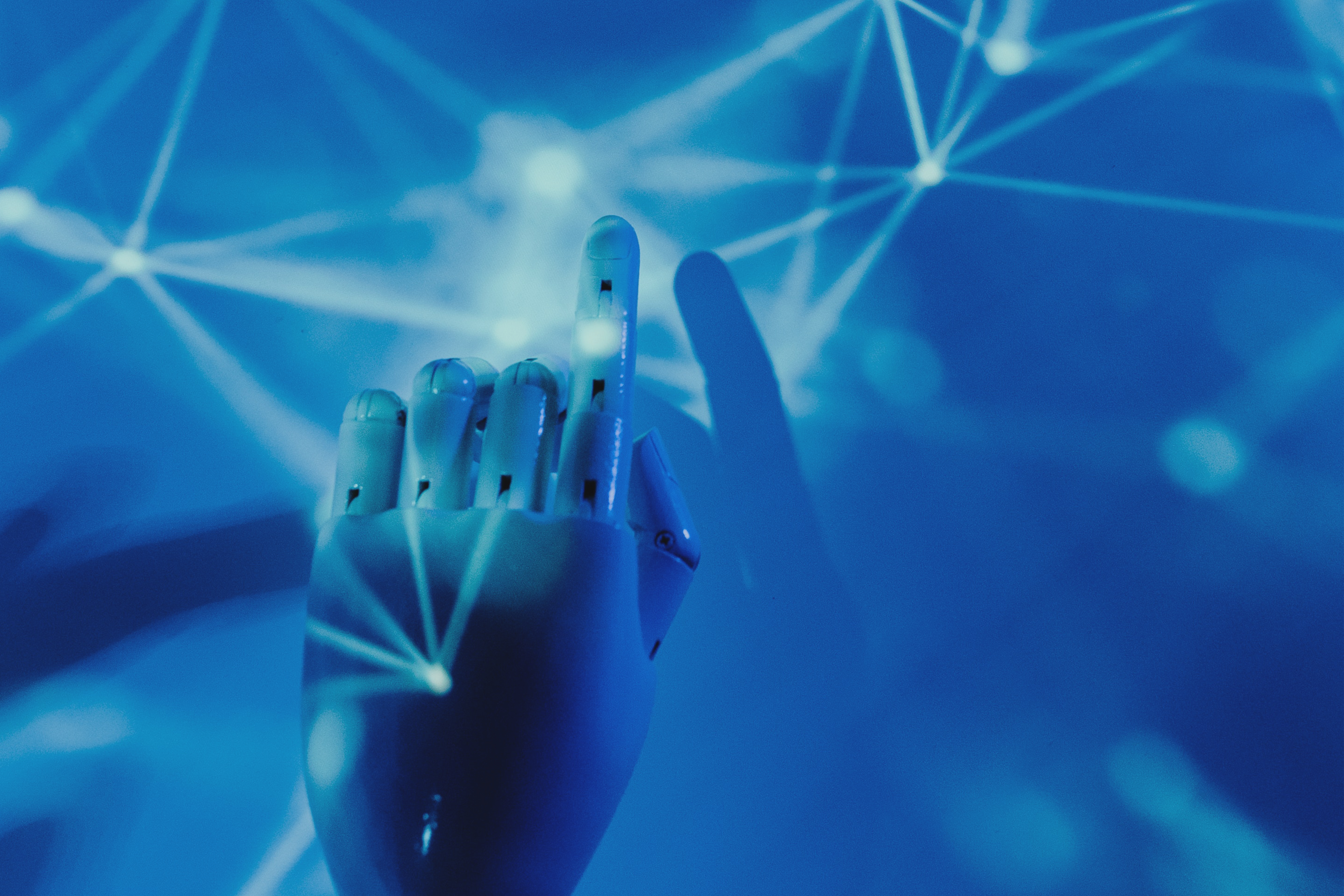
Abstract
This study investigates the integration of quantum circuits with classical neural networks for enhancing credit scoring for small- and medium-sized enterprises (SMEs). We introduce a hybrid quantum–classical model, focusing on the synergy between quantum and classical rather than comparing the performance of separate quantum and classical models. Our model incorporates a quantum layer into a traditional neural network, achieving notable reductions in training time. We apply this innovative framework to a binary classification task with a proprietary real-world classical credit default dataset for SMEs in Singapore. The results indicate that our hybrid model achieves efficient training, requiring significantly fewer epochs (350) compared to its classical counterpart (3500) for a similar predictive accuracy. However, we observed a decrease in performance when expanding the model beyond 12 qubits or when adding additional quantum classifier blocks. This paper also considers practical challenges faced when deploying such models on quantum simulators and actual quantum computers. Overall, our quantum–classical hybrid model for credit scoring reveals its potential in industry, despite encountering certain scalability limitations and practical challenges.
This paper was published in the Special Issue Quantum Computing Algorithms and Quantum Computing Simulators), May 2024.